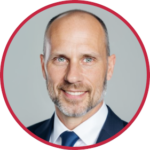
Although launched less than two years ago, ChatGPT has made artificial intelligence accessible to the masses, transitioning AI from nerds-only tech to a mainstream audience. The extremely fast adoption of ChatGPT has ushered in a proliferation of additional large language models with real utility to businesses and individuals alike.1
According to ChatGPT itself, its top 10 use-cases are currently:
- Customer Support & Chatbots
- Content Creation & Writing Assistance
- Programming & Code Assistance
- Education & Tutoring
- Language Translation & Practice
- Personal Assistant Tasks
- Data Analysis & Interpretation
- Productivity Tools
- Mental Health Support & Wellness
- Entertainment & Gaming
However, when asked about the top use cases for AI in general, not just ChatGPT, the answers were more sweeping.
- Healthcare & Medical Diagnosis
- Natural Language Processing (NLP) & Language Understanding
- Autonomous Vehicles
- Predictive Analytics & Forecasting
- Fraud Detection & Cybersecurity
- Personalized Marketing & Recommendations
- Manufacturing & Automation
- Robotics & Process Automation
- Financial Services & Algorithmic Trading
- Smart Cities & IoT
Adoption
For those delving into AI, 2023 marked the year where people began learning how to better prompt AI engines to get better answers. In the nascent AI period, which we are in now, smart input prompting is a real skill.
In addition to newer and smarter versions of ChatGPT, 2024 brought us additional general-purpose AI engines as well as “AI-for-something specific” applications.
The field of artificial intelligence has moved so rapidly, perhaps the best strategy for most businesses thus far has been to sit on the sidelines and wait for the dust to settle. Until recently, it has probably not been a good use of resources to build complex custom, in-house AI applications to solve difficult problems, especially if the development consumes considerable time and resources (which it would have). Odds are, about the time you completed it, the market offered something quite similar, but better, for free. Progress in AI is moving that quickly.
This was the strategic decision we made within our company, to grab the low hanging fruit of AI use-cases internally, but not to spend capital and resources to develop complex AI initiatives. In hindsight, I believe this was the correct approach.
However, developers and tech entrepreneurs have now built much of the needed infrastructure to more easily implement AI initiatives within corporate use-cases. We are now entering the season where the AI implementation scaffolding has been erected to allow companies to more efficiently construct AI solutions. Companies should now invest resources into AI endeavors for both internal and external initiatives to gain substantial operational efficiencies within the company and massive value enhancements to end customers.
Because of this…
We, collectively, are about to experience a colossal surge in global productivity for at least the next 20 years.
This will be much like the efficiency gained from the internet itself, (which enabled the development of AI), except, this wave may be on a larger scale. If that is difficult to imagine, hold on to your hat.
Even with all the talk of AI in recent years, this technology is still very much in the embryonic phase. The world is about to change so significantly, we will look back on today like the stone age, and simultaneously crave the simplicity we now enjoy.
What’s Everyone Going To Do?
People wonder if a lot of jobs will be replaced by AI. Yes, they will. Absolutely.
But this is the nature of technological progress. To fear this is akin to wanting blacksmiths forging horseshoes instead of driving automobiles; or reverting back to telephone operators manually inserting cables into switchboards to connect landlines fixed to the wall so people can talk by phone; or paying substantial amounts for “long distance” calls outside your area code (like the good old days), instead of making video calls on FaceTime around the world for free to a wireless device in someone’s pocket.
People are also wondering what’s left for humans to work on if AI and bots will do so much.
Here’s my answer…
Problems Worth Solving
AI is becoming quite good at answering questions and solving problems. But AI is not good at identifying the problems to solve. This skill still resides within the domain of the human mind.
Humans have innate desires, experience pain, annoyances, and frustrations. These problems, readily identifiable by humans, are worth solving.
It’s not clear to me that AI, in its current form, understands how to formulate a problem that is worth solving. I suppose it could guess at things, perhaps haphazardly, by combining ideas it already knows (adjacent possibles), but AI lacks the intuition to understand if the problem posed is worth solving. That is, some problems – most problems – we might construct have no practical utility.2
Left to its own randomness of problem identification, AI might try to solve for a purple donut slingshot. Let’s ask Google to show us an image of that…
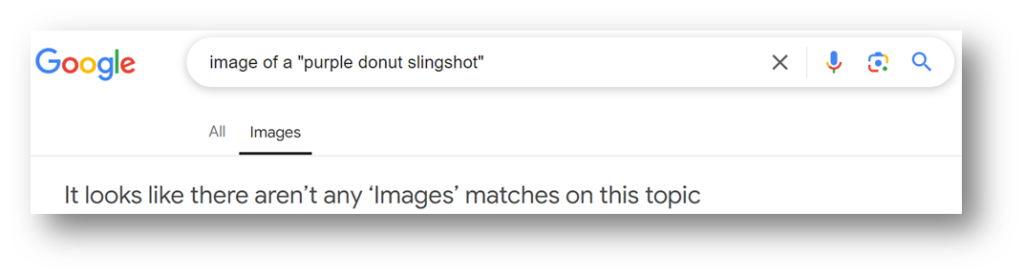
No images yet. Exactly. Nor should there be. Good job humans. No one in the world has made this. Until now…
I asked ChatGPT to create it.
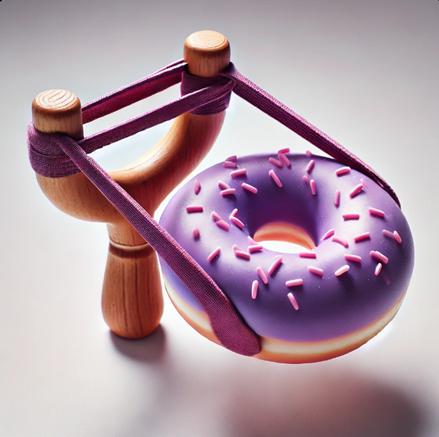
Good job AI, although I’m confident this concept has little market appeal or practical applications in the real world (or did I just throw away my best business idea thus far?).
Therein lies a problem with AI. In its current state, it responds to prompts, but lacks the ability to define a problem in which it might solve, lacks the ability to determine a worthwhile problem, lacks the ability to accept or reject a thesis on a definable problem set, and lacks the ability to determine if the AI solution actually proves to be valuable or useful.3
This AI deficiency is an opportunity for humans; to identify and frame problems that AI might help us solve more quickly, and likely more eloquently, and to determine if the solution works properly (or works as intended).
Future Skills To Master
Key human technical skills going forward will be the ability to:
- Identify and define a problem worth solving.
- Define the inputs and desired outputs.
- Communicate the problem and boundary conditions succinctly such that solutions consider real-world constraints.
- Integrate AI components to automate the ingestion of inputs and the communication of outputs.
- String together the [INPUTS] >>> [AI ASSISTED SOLUTIONS] >>> [OUTPUT] processes to form end-to-end AI-assisted solutions to large scale problems.
- Test the validity of proposed solutions.
Interestingly, this is exactly how we solve problems without AI. It’s just that AI will allow us to cycle through this process much faster while consuming fewer human resources.
AI, as a super-energy-efficient problem solver, will allow us to solve problems that currently exist, but are either too small to warrant the requisite energy/time/resources to solve, or too complex to allocate energy/time/resources to attempt a solution.
In the “too small of a problem” case, the limiting factor is an insufficient return-on-investment for the effort required to arrive at the solution. Stated differently, the opportunity cost exceeds the threshold ROI to proceed. It was a small problem that, today, we just deal with and work around, a minor annoyance. We have more important things to work on. Things with higher expected ROI.
In the “too large of a problem” category, the gating factor is “we may not be able to solve it without AI” because the cost to try is too high.
Of the steps shown above, Steps #1 & #6 might be the key human skills.
- Step 1 is crucial. Identify and define a problem worth solving.
- Step 6 remains strictly in the human domain.4 Test the validity of proposed solutions.
An Example
Here’s a fictious example to make the case for human intervention in Step #6.
Suppose we set AI the task of solving for “a higher growth rate of aquatic life in our oceans”. Sounds reasonable, even noble. Conservation of nature. All good so far.
One AI solution might be a proposal to kill all but the last 2 of each species such that the growth rate of 100% is now more achievable. It is much easier to move from two fish to four, a doubling of the ocean population. But this solution obviously misses the bigger picture and fails Step #6… probably because Step #3 failed to fully articulate the full set of guardrails and initial boundary conditions.
The underlying issue is that AI is indifferent to the solutions it presents, and the path required to get there. It has no innate preferences. It has no vested interest in outcomes. It’s simply a solution driver, regardless of the broader rationality of the solution provided.
Consequently, we must be careful how we define problems and how we describe the boundary conditions.5
Solving Complex Problems
Let’s probe further into the “too large of a problem” case to illustrate how AI might create breakthroughs in technology.
Let’s use the subject of Material Science to discuss an example problem that is entirely too complex for human endeavors, without AI assistance for the heavy lifting on the computational side.
But first, with apologies, we need to detour into some basic statistics to lay the groundwork and provide insight into the complexity of large numbers – to demonstrate how AI can help solve complex problems that we simply can’t solve today, due to a lack of computational resources combined with intelligent inference engines.
STATISTCAL DETOUR
Consider a deck of playing cards. If you take two playing cards from a deck, say the 2-of-hearts and the 3-of-hearts, how many unique ways can you arrange them (in a line… don’t get too creative on this)?
Simple problem. The answer is two different arrangements.
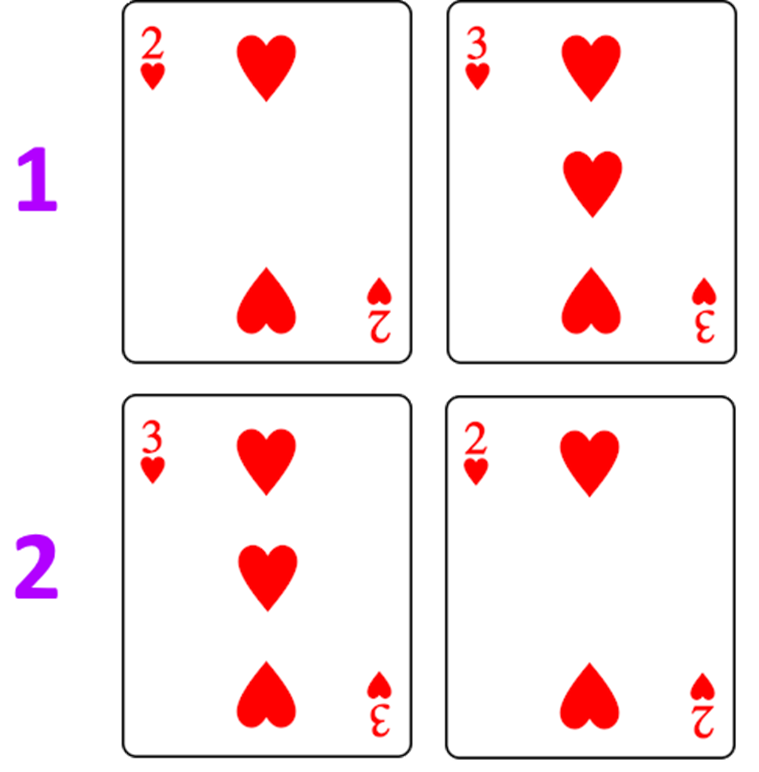
Mathematically, this can be written as two factorial, expressed as (2!):
2! = 2 x 1 = 2
Now, let’s expand our example to include three cards. The 2- and 3-of-hearts again and the 4-of-spades. How many unique ways can we arrange these?
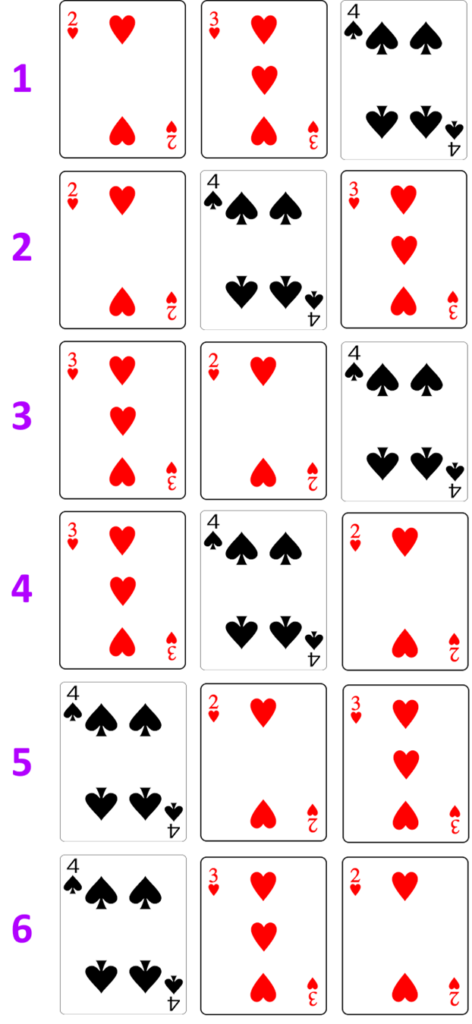
The answer is six ways, or three factorial (3!):
3! = 3 x 2 x 1 = 6
Let’s now apply the statistics to a full deck of playing cards.
A deck contains 52 unique cards, excluding the jokers. How many unique ways can you arrange all 52 cards? Following the pattern above, the answer is a quick calculation of 52 factorial (52!).
52! = 52 x 51 x 50 x 49 x 48… x 1
52 factorial = 8.07 X 1067
That is, a deck of cards can be arranged into this many unique combinations: 80,658,175,170,094,000,000,000,000,000,000,000,000,000,000,000,000,000,000,000,000,000,000
I wasn’t even sure how to read a number this big, so I asked ChatGPT:
80 quindecillion, 658 quattuordecillion, 175 tredecillion, 170 duodecillion, 94 undecillion
For convenience, I rounded to the nearest undecillion. That seemed close enough for our discussion here.
We can’t really get our head around a number this large, at least I can’t. So, let’s put it in more practical terms.
If 10 billion people (more than the current population of the Earth) each shuffled a deck of cards (that is, 10 billion decks of cards) every second of the day, 24 hours per day, 365 days per year, for a billion years, they wouldn’t even get close to half of this number.
The practical implications here are astounding. That is, every time you shuffle a deck of cards, there has likely never been a deck of cards in that order before. Ever.6 And when you shuffle the deck again, once again, that specific card arrangement has almost certainly never happened before… to no other deck of playing cards anywhere in the world, in all of history, ever. Nor will it ever happen again. It’s just so statistically improbable that we can say this with a high degree of confidence.
So, why does this matter and what does it have to do with AI?
Back to AI and Complex Problems
Consider the products we have invented thus far in human history, products made from materials where we haphazardly combined certain atoms and molecules together in ever-increasingly clever ways to devise new materials that did not previously exist. I’m talking about products made from stainless steel, plastics, ceramics, carbon fiber, Kevlar, etc. These are complex materials not found in nature.
Cool. But how many combinations have we really tried, relative to the possible number of combinations that could be tried?
Well, there are considerably more elements in the periodic table than the number of cards in a deck of cards. How many elements? Between 91 and 98, depending on how you define “stable”.
The various combinations of these results in massively more potential combinations than a deck of playing cards… and this assumes each element is mixed in a 1-to-1 ratio. To add to the complexity (and the quantity of potential combinations), we can also mix each of these elements at different ratios, including zero… meaning some elements could be excluded from the recipe. 0% is the most common quantity for the 91 elements in all existing products made today.
With the playing card stats, we assumed the deck always remains 52 cards. The number of combinations goes up considerably if we also allow for deck combinations with only 1 card, or two cards, or three cards, up to 52 cards.
And, we didn’t even talk about the process of combining things. For example, elements may be combined at different temperatures, at various pressures, in a variety of shapes, in different orders, mixtures vs solutions, etc. Each of these add layers of complexity to the final outcomes.
All that to say, there are literally limitless combinations of base elements that could be combined. There are not enough human hours to try even the smallest fraction of possible combinations. It’s truly amazing we have discovered as much as we have thus far, given the sheer number of endless possibilities. We are not even at the Neanderthal level of material science.
We have materials today that would get you burned at the stake if found in your possession several hundred years ago. Imagine what we have yet to discover.
There are materials out there that AI will identify as potential candidates with properties that will change the world and allow for new products and services that previously did not exist.
Very recent innovations in human history have propelled travel velocity from horseback to jet engine to rocket boosters, in part through innovations in material science (materials that can withstand enormous ranges in temperatures and pressures, for example).
AI has the capacity to simulate properties of materials in various combinations and produce a list of potentially useful materials never previously considered… and certainly not tried in the lab yet. Furthermore, AI can simultaneously discard combinations that have less obvious value (so far).
Rather than have humans create materials in the lab and test, one-by-one, AI on a massive server network can “invent” new materials at several trillion times the speed compared to what we do today manually.
There will be materials that are stronger and lighter than anything we have today.
There will be materials that exhibit lossless electricity conductivity.
There will be materials that are invisible.
There will be materials that produce a steady source of localized, safe heat (or power) for decades.
There will also be materials that make modern chemical warfare seem like fart spray in comparison. (Of course, this is a potential downside risk of technology).
The point of this discussion of advancements in material science is just to show how transformative AI might be, just in this one domain. This discussion has only been about material science. How many other problems are we not currently solving due to a lack of resources or because we are currently working at snail’s pace compared to AI? Lots.
Statistical Truth: we think we are modern human beings, but of the things we might know,
Almost everything remains unknown to us today.
The larger point is this. There are…
No Shortage of Problems to Solve
With AI, we can now solve for problems that we would not assign to a human because it would require more energy than it is worth, if a human had to perform the task. But with AI, the energy-to-solve (equivalent to the cost-to-solve) decreases so much, we will now assign AI to solve both enormous-scale and miniscule problems for which we previously did not even seek a solution.
Consequently, many things will become incredibly optimized, as we eke out the last 0.1 percentage points in process inefficiencies that currently exist.
Here’s an example from my own company.
A SPECIFIC EXAMPLE
I founded an information services company in 2005. We work with data. Lots of data. Data pairs with artificial intelligence. You can’t have AI without data. But AI can also make your data much better and help the company operate more efficiently – higher product quality, faster update cycles, lower cost. Consequently, we have incorporated elements of artificial intelligence into our operations.
There was a set of additional information we wanted to add to our product. However, the resources to implement this were previously unaffordable, given the benefit. That is, it would take considerably too much time (and therefore cost) to produce this new data and keep it updated, compared to the perceived value the data has in the marketplace.
For this specific project, five years ago, I’d estimate this would have taken 25 – 35 human researchers two years to complete, working full-time, and perhaps 20 full-time employees to maintain the data quality into perpetuity. In other words, it would have been cost prohibitive, relative to the estimated benefit for our customer base.
By contrast, recently, one of the members of our existing team solved this using AI, working ~25% of his time on this project for perhaps six months. Further, on-going maintenance is probably the equivalent of 4 weeks of a person’s effort each year. In other words, the efficiency gain (or cost reduction, in this case) is one full-time equivalent person for one month per year (~160 hours per year) compared to 30 full-time people for two years ~124,800 hours per year). That’s 0.1% of the required human effort, a 99.9% productivity gain… and I’d estimate the quality of the output is nearly equal, maybe better.
I suppose it’s not fair to place a percentage on the productivity gain, because, without AI, this project would never have existed, and our customers would not have benefited from this new data as part of our product offering.
Further, we did not have to review 1,000 applications to interview 200 people, such that we could recruit 30 employees to do the work. Nor did we have to hire a project manager to oversee it all. And, the time to implement went from 3 years to six months (and it was only six months because our data scientist was only allocating a fraction of his time to it, working on it in the background).
This level of productivity gain is a common occurrence with AI.
AI will infuse extreme cost efficiencies into the workforce, which will trickle through almost every product and service within every industry.
I wrote about this previously in mid-2023 with a blog post titled:
The Imminent Transformation: How AI Will Revolutionize Industries and Reshape the Workforce
Adoption & Disruption
So, here’s where we are right now, as best I can tell.
AI tools are sufficient to reduce costs, add efficiencies and blossom thousands of new products and services, the likes of which we have never seen before.
What’s the hold up? Human adoption.
The average business leader has heard of AI, but they are not yet actively implementing AI strategies in their companies. This is mainly because they do not know where to start.
Some companies, the minority, have an idea on where AI might help them, but they do not have the technical expertise in-house to implement AI solutions and infuse it into their workflows and product offerings. However, the strategic advantages of AI are already so powerful, we are about to enter a season where some companies within each industry vertical will adopt AI technology and gain efficiencies while other companies in that same vertical will simply not be able to compete.
Some companies will use AI to disrupt, and many companies will be disrupted.
For industries without an AI champion, emergent companies will enter the space and topple the incumbents.
Conclusion
There will always be work for humans to do, but the shape of that work will change, as it always has across time and will continue to do in the future. Our primary technological role will shift to define worthwhile problems, to devise methods for AI to propose potential solutions, and to test those solutions against their real-world viability.7
AI will massively change the way we solve problems and will disrupt the way we do business. Nearly every facet of life will be touched by AI as it ushers in a new season of fast-paced change and flux.
Existing companies need to define how they can infuse AI into their operations, products and services and begin to work on that as a key initiative beginning in early 2025.
Through AI, we are about to experience and uncover some truly amazing, unimaginable discoveries. Today, some high school student is tinkering around with AI in the late hours of the night on an idea that will eventually revolutionize an entire industry or birth a new one.
It’s an exciting time to be alive, even if uncertain.8
Follow Past Midway if you would like an email notification of new posts.
FOOTNOTES:
- Except for the lists below, this article was written entirely by me, as an original thought piece, and NOT generated by AI itself… because that would be rather circular.
- Our brains behave the same way. The brain is an idea generator. This does not mean that all ideas presented to us by our brain are good ideas. Most are not. An efficient mind quickly rejects bad ideas to free resources, capacity and time to consider better ideas in more depth. AI, in its current form, lacks the ability to reject bad ideas or recognize good ones.
- The astute reader with a keen eye might also notice that the slingshot, as drawn, wouldn’t shoot anything well. For some reason, it has the strap bridging the two forks, blocking the projectile’s path. Further, how is the donut is attached to the sling? I would also appear that the donut is wider than the slingshot’s fork. For all these reasons, this is a bad design. Although it is a potential solution and the donut looks tasty, clearly, the slingshot would not work as constructed in the image. This is indicative of the early stages of AI we are currently in. This will improve with time.
- At least for now. In fact, one of the challenges of developing ever-increasingly more powerful AI systems is the resources required for human experts to test and validate/verify the veracity of the solutions provided. At some point, the solutions AI proposes will exceed the best-in-class human experts in that field. And that is precisely the point… that AI will begin to arrive at solutions to highly complex problems that world-class industry experts have not previously seen. This is the leap forward.
- Of course, this is nothing new to problem solving. Human-suggested solutions experience the same challenge. For example, adding corn-based ethanol to gasoline to reduce the reliance on hydrocarbons from drilling as a fuel source arguably had a net negative outcome. Sounds good in principle, but one outcome was the burning of the rainforest to create more fields to grow more corn, given the profitability of corn with the increased demand for the commodity. This is an unintended consequence. There are many examples like this. It stands to reason that AI would make the same mistakes, as it is developed by humans, with human inputs as its fundamental operating parameters and foundational base-knowledge. Its current fundamental flaw is that it has been trained by humans, which includes all our specific and collective biases and faults.
- Assuming it’s a random shuffle and you are not manually putting the cards back in order.
- Interestingly, and worth noting, non-technical problems remain more constant through time. We still need love, friendships, relationships and connection. AI will not solve for these. In fact, AI will likely hinder these components of life as it begins to simulate a less-rich, less dynamic, faux-approximation of these relational elements of life, especially for our youth.
- If you managed to read to the end here, you know I indeed wrote this entirely myself, because AI would have written it more succinctly… but AI would not have produced the problem set of its own limitations as part of the article.
Fantastic read. Being the geeky family we are, my crew has lively debates on this topic. Can’t wait to forward this to them. Thanks as always for sharing your thoughts with the world.
Fascinating, as always. Coming from one who plugged in those telephone connections making possible the communication with those wall phones, the consternation is real, while, at the same time, the feelings of optimism with the advances in human health which are, no doubt, already being made (to mention only one area) are also strongly felt. The other thought was mentioned in footnote #7. Those “relational components” are already being eroded with the extensive, pervasive cell phone usage. Lastly, “fart spray”?! Good job yet again. I hope you are grateful for your dad’s incredible intellect.
AI has certainly become a hot topic and it’s definitely becoming one if the most transformative technological developments of our lifespans. I am interested to see how we as humans develop it and choose to implement it in both business as well as our personal lives. I do think generative AI will develop even past what you’ve outlined here and will eventually be able to ‘prompt’ itself. My final paper in my college robotics ethics class covered artificial mimicry of living creatures and sentience and I took the stance then, as I do today, that what we know as ‘science fiction’ is really ‘science future’ more often than not.