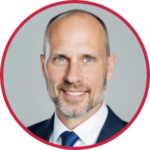
I am continually intrigued by how two groups of people can witness the exact same event – either live or on video – and arrive at completely opposite conclusions. This happens most frequently with sports and politics… from parents at little league to stadiums of fans at pro events… from homeowner associations to federal politics.
While the perspective can be different between two observers at the same live event (this can be important), with video, the perspective is the same. In this case, to arrive at opposite conclusions, one side, or the other, or both, must imbue some preconceived notions upon the event. That is, the event is filtered by, and intertwined with, our preconstructed beliefs and opinions.
The odd thing is, we are quick to accuse everyone else of doing this, but we rarely consider we are simultaneously doing the same thing. Surely, we aren’t ALWAYS right. Surely, everyone else isn’t ALWAYS wrong.
A telltale sign that we are deceiving ourselves and unaware of our own biases is the unwavering belief that we are right most of the time.
The truth is, we don’t always perceive events exactly as they happen. The audio-visual experience arrives to us pre-filtered, by us, unbeknownst to us.
Background
Building on my 2019 article, Mistaking Noise for Signal, this long-overdue extension explores how we construct False Narratives… and believe them… and actively seek corroborating evidence to further substantiate what we already trust to be true.
I previously wrote:
“The problem with these errors in our thinking lies in the stories we tell ourselves – completely fabricated stories contorted to fit observed data. These stories represent our model of the world for the data we have seen. But they may have very little predictive value.”
Our mental models are only as valuable as their ability to predict future outcomes, regardless of how well they align with historical data. In the words of Gordan Livingston:
“If the map doesn’t agree with the ground, the map is wrong. [ ] …our passage through life consists of an effort to get the maps in our heads to conform to the ground on which we walk.”1
Let’s Talk About Data
My company works with data. Lots of data.
Looking back across my career, every position I’ve held, starting with my college internships, has involved structuring and analyzing data in some capacity. Why? Because I unknowingly bent my projects in this direction. It wasn’t until much later that I realized I naturally gravitate toward structuring data, uncovering patterns, and teasing out the stories they reveal – or, at least my interpretation of the stories (a key difference).
I should have identified this personal inclination much sooner, which brings me to a side point.
Your History Illuminates Your Path
Young people would do well to reflect on what has naturally drawn their interest and engagement in prior work or school projects. Our natural inclinations aren’t random. These motifs are signals, quietly pointing us toward where we might find fulfillment.
The patterns of our past pursuits often reveal the clearest guideposts for the most promising paths ahead.
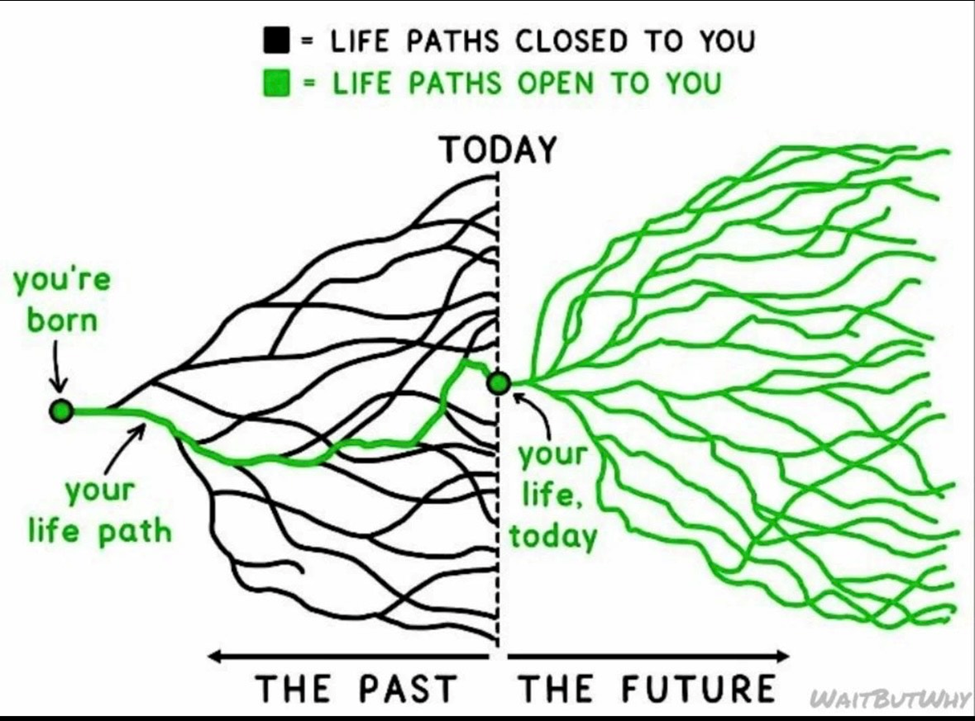
Credit to the Wait But Why blog for this excellent visual.
Start with Raw Data
With decades of experience, I am now predisposed to chase data back to its source, in its rawest form, whenever possible. Unaltered, original source data is the best place to start thinking.
Secondhand (manipulated) data, invariably contain biases, even if inadvertently. Summarized data is lossy, by definition – a compressed and filtered version of its former self.
Unless you are working with the original source data, assume someone has monkeyed with the data.
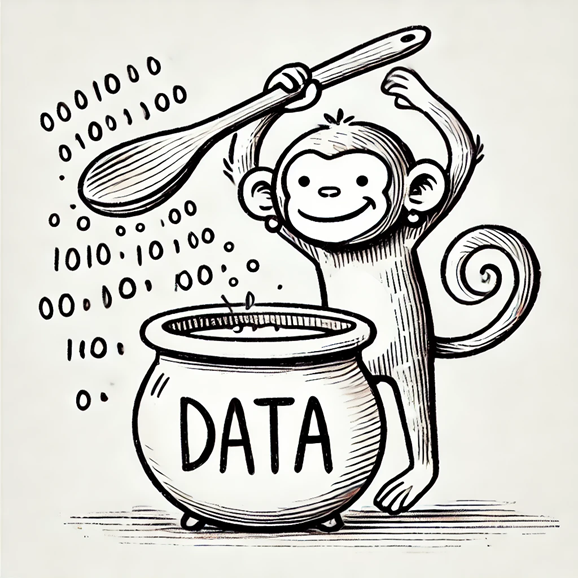
Even with raw source data, we must understand the data gathering methodologies, consider the statistical significance of the sample size, question hidden motives, and explore other factors that might bias the data. Consideration of these additional dynamics help bracket the degree of confidence we might place on the predictive quality of the underlying data and its representativeness of reality.
Data Quality & Reasoning
When data point to a conclusion, it’s easy to mistakenly assume the conclusion is the implied truth, “because the data proves it. It’s science.” But we frequently force the wrong narrative onto the numbers. The table below shows the data/reasoning quality categories:
Faulty Reasoning | Good Reasoning | |
Bad Data | Complete nonsense. | The only logical conclusion for this category concludes the data is bad. Nothing else makes sense here. |
Good but Incomplete Data | False narratives. | Tentatively useful models, if recognized as limited, special case scenarios. |
Good, Complete, Unbiased Data | False narratives amplified by over-confidence. | When you think you are in this category, the ONLY reasonable assumption is that the data are likely incomplete or biased in some fashion. See above. Yet, this is the best starting point for good mental models. |
Assuming the data is sufficiently complete, unbiased, and accurate, (although it probably isn’t), and assuming we apply reasonably good reasoning to it, there are still potential pitfalls. Let’s discuss some of the common ways we can still get this wrong.
Selection Bias
Sometimes, the data isn’t all the data.
Consider this claim from an insurance advertisement:
“People who switch to ABC Insurance save 20% on average.”
Let’s assume this is in fact truth-in-advertising and they have the data to support this claim. The implied assumption is that ABC Insurance somehow provides cheaper rates, compelling people to switch carriers. Listeners of this advertisement often extrapolate this to mean, “I should consider switching my insurance provider because I might also save 20%.” This is the conclusion the advertiser wants to elicit. It’s probably effective.
But there’s a selection bias in the data.
Of course, people save 20%! Otherwise, they wouldn’t switch, would they?
Those who stood to save ~20% actually switched to ABC Insurance. Those who already had price competitive insurance, or perhaps even a lower premium, did not switch to ABC. This is common sense, buried in the nuances of the statistics.
This is selection bias, in that the outcome and inferences are based on a specific slice of data… in this case, on purpose.
Survivorship Bias
Survivorship bias is akin to selection bias, but perhaps not as overtly nefarious.
Consider the following statement you have almost certainly heard:
“A survey of people over 80 years old revealed their biggest regret in life was not taking more risks.”
The underlying data supporting this statement contains a survivorship bias (assuming there is actual data for this… let’s ignore the idea that maybe someone just made this up). The people surveyed, now in the later stages of a long life, were eligible to take the survey precisely BECAUSE they were still alive at 80+. Could it be that the very reason these people lived to be 80+ years old is that they took FEWER risks in life?
If the survey included people who died at a much younger age, might these people list their biggest regret as “taking too much risk”, or maybe, “taking that one big risk at the end”?
Survivorship bias skews the data. If we fail to identify it, we risk telling the wrong story. In this specific case, we are supposed to infer we should take more risks in life – to live life to its fullest. Or maybe not, if we would like to live to 80.
Here’s another example of survivorship bias, but on the negative side:
Many of us have a deep-seeded impression that criminals are idiots. YouTube alone can provide a near-endless stream of proof. However, almost all our data on criminals come from those who were caught. It’s safe to infer these criminals were not (on average) as clever as those that got away with their crimes.
We don’t refer to those who got away with it as criminals. We call them citizens, blissfully unaware of their covert criminal activities.
This is a case where our data are clearly biased, limited to a pre-selected sliver from one side of the equation. Criminals (on average) are likely much smarter than our general impression of them.
Correlation vs Causation
Sometimes we have “good” data but incorrectly assume correlation implies causation.
Most of us have heard this statistic:
“Most car accidents happen within one mile from your house.”
Wow! It must be dangerous to drive around my house, and yours. Logically, it might be safer for me to drive around your house, and for you to drive around mine (assuming we live more than two miles apart). Perhaps you should run my errands, and I should run yours. Think of all the lives we could save!
Statistically speaking, most trips start and end at our house, regardless of how far we drive. Consequently, we frequent the critical one-mile radius on most trips. Probabilistically, most of us are likely to be one mile from home, more than any other place. Hence, the skewed reasoning from this statistic.
Substitutional Risks
As humans, we are particularly sensitive to risks, both real and imagined (although imagined risks plague us more frequently). Sometimes, we fail to understand when risks are mutually exclusive or additive.
A friend once told me he was going skydiving.
“I wouldn’t want to jump out of a plane,” I said.
“Why not?” he asked.
Curious question. I had assumed the burden of proof was on him to rationalize why he would jump out of a plane and not the other way around.
“Because it’s risky,” I said.
“Skydiving is statistically less risky than driving your car,” he informed me.
Let’s assume the data corroborates this statement (I’m uncertain on this point, but let’s make this assumption). The fallacy here is that these are somehow mutually exclusive risks. As if the risk I assume when jumping from an airplane somehow reduces, replaces, or negates some of the risk I incur by driving. The reality is, by skydiving, we are now incurring the risk of skydiving AND the risk of driving. So, these risks are additive (unless I just stop driving as soon as I start jumping out of planes).
The irony is, we must drive to the small airport to jump out of the plane. And, I’m pretty sure we’ll also pass through the most dangerous area on the way, less than a mile from the house! Good grief! Risks piled upon risks!
It’s probably also statistically safer to stand in the middle of a field during a lightning storm in Oklahoma than to drive on a major freeway, but that doesn’t mean it’s a good idea.
Although these are similar comparisons, no one uses the standing in a field during a storm argument to comparatively scale the risk of skydiving. Why? Because standing in a field is not fun. Skydiving is. Consequently, people murmur this statistic to themselves to muster sufficient gumption to jump out of a plane. This is most likely the more accurate narrative at play here.
Data & Stories
Data tell stories.2 But it’s easy to construct false narratives from data. This most often occurs when we do not recognize lurking statistical biases buried in the data or ignore the propensity of humans to use data to narrate a preconceived notion from a particular slice of data, or from incomplete data.
It is considerably more difficult to reason from first principles. That is, from raw data >> to thesis >> to narrative. We are much more inclined to work this equation in the opposite direction, which is expeditious, but not intellectually rigorous.
People are wired to contrive stories from observation.
But in doing so, we create reasoning errors.
Reasoning Errors
Here are seven reasoning errors we fall prey to that distort our interpretations of data and obscure our ability to construct a proper view of the world:
1. Confirmation Bias
We all carry within us an inclination to accept certain narratives first and then seek out data that aligns neatly with our preconceived beliefs. It’s so natural, most of us fail to notice when we’re doing it.
The primary psychological reason we narrate is not to discover something new, but to fortify our previously held beliefs.
By prioritizing confirming evidence and downplaying contradictions, we simplify complex decision-making by reinforcing preexisting mental models rather than challenging them.
The problem is, once we solidify a certain idea of how we think things work, or what we believe to be true, it can be very difficult to re-wire that circuit to change our minds. It’s admirable, but unlikely.
Changing a fundamental view is a mentally anguishing process (initially), but ultimately, it can be profoundly liberating.
Sometimes our greatest freedom lies in the humility of acknowledging how little we truly understand.
2. Cognitive Dissonance We all experience a certain psychological discomfort when confronting data that conflicts with our closely held beliefs or assumptions. To reduce discomfort, we tend to reinterpret, downplay, or dismiss conflicting data, reinforcing a familiar narrative. This seems simple-minded, but we all do this to varying degrees.3
The real problem arises when we assume we are less guilty of this than others. This mindset may indicate we are blind to our own cognitive dissonance.
During my research for the blog post titled, Where to Cut Federal Spending – An Economic Plan for the U.S. – Part 2, I downloaded and reviewed raw data on tax policy changes and studied their impact on GDP growth. I was mostly looking for supporting data to more officially confirm what I already knew to be true. My prior understanding had been shaped entirely by what I had heard from sources I had trusted as authoritative on the subject, layered with my own assumptions. I had not previously reviewed the raw source data myself. But as I examined the data, I had to do a double take. It seemed to show the opposite of what I thought.
Even though I am keenly aware that I can be wrong, I must admit, my first inclination was to check if the data was wrong (not a bad action to take, but interesting that it was my first inclination). Upon discovering the data was in fact correct, I then tried to argue (with myself) for some special case where I could still be right. I sliced and diced the data a few times, a few different ways, (within reason so as not to completely fool myself), to see if I could coax out a different conclusion. Eventually, I had to admit that I had simply been wrong. My incorrect beliefs had clouded my knowledge of economics on this point. Consequently, that same blog post included the following paragraph:
…when I started writing this post, I assumed the data would prove my previously held opinion, and this would be just a more rigorous analysis to show what I already knew to be true. But, to my surprise, the data showed otherwise. So, I need to admit to myself that I was wrong on this point.
But notice, I wrote “admit to myself”. Clever. I’m pretty sure I did not return to prior conversations with others of dissenting views on this point to also tell them I had been wrong. Fortunately, some of them read my blog and were vindicated more quietly, without even leaving a “I told you so” in the comments.
3. Narrative Fallacy
The human brain is inclined to make sense of complex data through familiar patterns rather than objectively evaluating the full complexity from a blank slate. Most of us intuitively favor coherent stories or explanations because…
Narratives simplify reality. For this reason, our narratives are NOT reality.
Sometimes, the overly simplified narrative we tell ourselves, (or allow ourselves to believe), is inaccurate.
4. Anchoring and Framing Effects
We tend to anchor our interpretations to initial impressions or ideas, shaping how subsequent data is perceived. Once a narrative takes root, it becomes difficult to reinterpret newly ingested information neutrally. Shifting our perspective to the other side of a viewpoint is unusual, especially in more volatile topics like religion and politics.
5. Cognitive Efficiency and Mental Shortcuts
Thinking from first principles is mentally taxing and requires deliberate effort. Relying on preexisting narratives or heuristics reduces cognitive load, enabling quicker decision-making, which can be important, but we should recognize that it can be at the expense of objectivity.
6. Motivated Reasoning
People have emotional, financial, ideological, or reputational incentives to support certain narratives. These motivations encourage selective interpretation of data to reach desired conclusions, rather than neutral or unbiased analysis.
Sometimes there is “a story” – the one you are told… the official narrative – and then there is “THE story”, the one more akin to the truth. An excellent method to tease out “THE story” is to follow the money. The potential for wealth and power corrupts narratives in the marketplace of ideas. This is motivated reasoning.
7. Social Identity and Group Dynamics
Individuals often align interpretations of data with narratives accepted by their social, cultural, political, or professional groups. This tribal alignment strengthens group cohesion and social belonging, but almost always compromises objectivity.
Tribalism and group-think are significantly amplified by the rise of social media, where we go to simultaneously indulge in our echo chambers and despise voices of opposition – both are engaging, as social media platform owners and optimizers have learned. We stay longer, and consequently watch more ads, as we are conditioned to both love and hate each other.
If we are not careful, we might find we have grown to love those we do not know and loathe those we love most.
This particular reasoning error is so divisive, it may have been the downfall of many societies historically, and the bane of ours today.
To ensure we are not contributing further to the negative societal impact of tribalistic thinking, let’s consider the following barometer:
Repeating phrases we have heard elsewhere, verbatim, without our own independent study and thought, is a good indicator we have surrendered too much of our thinking to the influence of a tribal identity.
Recognizing these reasoning errors exist helps us identify them in others – and, more importantly, in ourselves. We are all, to some degree, shaped by faulty thinking. Acknowledging this quiet self-deception is the first step toward greater clarity, enabling us to confront the psychological, cognitive, and social biases that influence our perceptions and inform our thinking.
Bonus
Don’t waste your time arguing with others when they make statistical fallacy errors like the examples above. You won’t change their mind. You won’t alter their narrative. It’s already locked in. They’ll just think you’re a jerk. And they’d be 63.7% right, 95% of the time, statistically speaking. Don’t question it.
But then again, I might be wrong…
Related posts:
My Father Knew He was Fallible
Follow Past Midway if you would like an email notification when I post something new.
FOOTNOTES:
- Too Soon Old, Too Late Smart. Gordon Livingston. 2004.
- I know, “data tells stories”, sounds better. But “data” is plural and should therefore take a plural verb. “Datum” is singular. But writing “Datum tells stories”, just sounds goofy. This article includes different variations of both. We’ll agree to look past that inconsistency.
- Do you know someone like this? Do specific names come to mind? Now be honest… did you consider and evaluate yourself? Exactly. Don’t worry, I didn’t either… and I wrote it! Guilty as charged.
Crazy how we all think we’re seeing the full picture, but really just picking out what fits our own story. Great read!
Thanks for your comment Rea!
Great article as always!
I’ve always been very interested in the study if how people think, including logical fallacies, decision making, etc. There’s a lot of good information on the topics online of anyone wishes to learn more.
I will comment that i disagree with saying not to argue/debate with those who fall ill to these fallacies in their reasoning. Many times, challenging that fallacy (in the right way) can be precisely what helps someone look inward and grow as a person and as a rational thinker. We should also all constantly strive to be doing this ourselves to help guard against biased or unhelpful trains of thought.
David – thank you for reading and for your comment. Great feedback. I actually agree with your pushback on the debating with others… the last section was intended to be somewhat humorous. I suppose the key on this point is to know when parties are receptive to other viewpoints and when they are not… and to know when we are receptive and when we are not.
Great article. I always appreciate your insights.
Thank you!
Very interesting, especially, number “7. Social Identity and Group Dynamics”. I think we all find ourselves guilty of these charges. I will try to identify such activity and correct myself in the future.
Thanks again for your thoughts.